Several months back, I wrote a blog about considering the implications of a cloud provider data quality (DQ) solution. This blog received great feedback, but it presumed that organizations were seeking to buy data quality solutions and not just build their own. Some readers asked, “what about just building my own solution? I have developers and a budget.” Some had even seen vendor DQ solutions and said, “well, I can build that myself.”
Reflecting on that feedback, I would like to share some of the implications of building your own DQ solution vs. a Commercial-off-the-Shelf (COTS) solution. I hope to explore both options in detail.
To refresh our last discussion, we focused on a couple of key themes. Those themes considered tech resource quality and organizational complexity. Underlying those themes, the blog quantified the fastest “DQ Time to market” as your strategy when making “buy” decisions. Our research proved that you should consider a DQ offering from a data intelligence company instead of a point DQ offering.
I believe the same concept could be applied to “build vs. buy” as well. As a leader, who cares for Data Quality and Observability, you want to prioritize DQ time-to-market when considering your technology. And how it will scale to ensure the least amount of risk associated with bad data.
Find out how Froedtert & the Medical College of Wisconsin used Collibra to improve data governance and make quick, effective data-driven decisions.
Read their customer story and discover what we can do for your organization.
1. Technical resource quality – Can you afford to build and maintain?
Most companies today are tech companies. This is seen in the words like Fintech, Medtech, and even ‘true tech.’ Further, companies are now starting to call themselves data companies. These trends correspond to the proven return on investing in technology and monetizing data. But while companies seek to innovate in the scope of their industry, leveraging their data, not every investment will yield the same returns.
Product organizations would find great value in digitally transforming a key aspect of their front office, tying the data to a centralized store, and leveraging analytics to beat the competition. But while they build on those features, they would not likely “reinvent the wheel” with applications like Outlook, JIRA, or Salesforce. Industries like Financial Services, Healthcare, or even Energy would either leverage those applications or look to their own industry competitors.
The main driver is the sunk cost. An organization can build an email system, but would look at all the additional resources necessary to re-create something that Outlook or Gmail already accomplishes. As history shows, some companies tried these decades ago, but the industry-driven application prevailed.
Consider that analogy alongside the build-DQ financial implications chart below. The below chart shows some real additional costs for building a home-grown DQ solution. Each resource represents something different from core-organizational data functions like pipeline, ETL, or analytics. In my personal experience as a DQ product owner, I have seen data product builds take years – not months. Could you justify the below cost? If not, would you then be hiring less than adequate resources, failing to even deliver a ‘working software’?
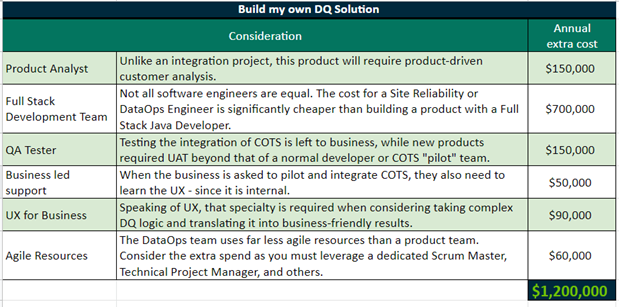
Figure 1. What would it cost you to BUILD a DQ solution?
If it is proven difficult enough to build, imagine then the time to maintain data quality. Almost immediately, any organization that transitions from build to run has to make tough resource decisions. In many cases, the team that builds such a product finds themselves questioning their role in a run mode. What allocation goes to new product features vs. maintenance? Do our best resources leave?
While this product team struggles to become a data operations team, two factors remain constant. The organization will change (see the next section), and the competition will increase. Yes, you build the perfect product today, but can you keep up with the COTS of tomorrow? Especially when those offerings are solving unforeseen challenges your organization has not faced? Imagine if your CIO suddenly announces that new cloud migration requires new features and you’re already behind schedule?
Would every new refactor, feature, or upgrade cost as much as the previous projections? Maybe! At the very least, consider the below graphic in comparison to a SaaS/Cloud DQ offering. Upgrade installations alone can cost you ~$70K annually. Those resources and money far exceed the typical vendor “hosting” cost of a COTS DQ product.
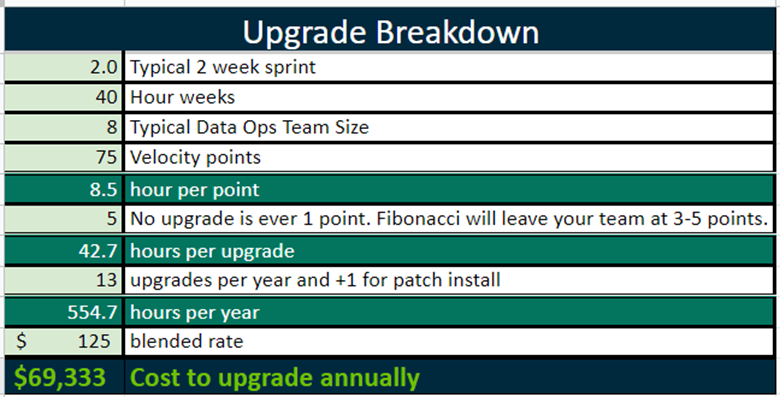
Figure 2. What would it cost you to UPGRADE a home-built DQ solution?
2. Organizational complexity – Can you really out-technology your organization’s people and process?
A critical reader could cite exceptions to the above data, recognizing that a good anecdote challenges facts. The anecdote is often a great way to qualitatively disrupt quantitative analysis – typically prefaced with a “we’re different…” As a former DQ product owner, I welcome that challenge and probably acknowledge its truth, but I would posit that the sum of those anecdotes does not outweigh the greater cost-benefit analysis.
Perhaps we could consider some of the challenges relative to organizational complexity.
- We’re different. We pay people to build data products – Yes, I was paid first to build financial products, then data products for financial services. The industry-focused product was necessary and fulfilling – and I am personally seeing a different passion in data products being built at a data company. As a product owner, my tradecraft is products, customer journey, and agile, not just data governance. So I would challenge that your data product teams would be just as effective at building your industry applications.
- We’re different. I can build this myself – Yes, I believe that a super team of ultra-high performers can often build great technology. The underlying tech behind Collibra Data Quality & Observability was built by a team of 20 people. But how long does a great team stick? Will these super performers continue from build to run, or move on to their next collective initiative? In this case, I would challenge the team’s leadership. Do you think you will be able to afford that same group when they realize they have monopolized your organization’s data needs?
- We’re different. Our data is too complex – Yes, customized architecture is a driving force behind data enablement, governance, or intelligence. The customized architecture was a source of problems inhibiting automation and digitalization initiatives like moving to the cloud. Nevertheless, while your organization may be complex, you are not the only complex organization. A good COTS solution will get you 80% of the way there, leaving you to only build for the last 20%. A key theme in any complex organization is to build to the target state, not fight the tides of the current state.
- We’re different. We’re highly regulated – Yes, a highly regulated organization experiences great scrutiny in its proposed solutions. While we could debate the nature of the audit, we all agree that not every audit is equal. Auditors are trained to assess risk and name brands that alleviate risk. I would challenge that an audit on Gartner-rated technology would receive less scrutiny than a non-data industry’s attempt to compete.
- We’re different. We’re too political – Yes, office politics drives many decisions. These decisions revolve around negotiating perceptions around people and processes. Office politics are subject to pressures beyond facts, and I would challenge that industry politics trump office politics. Every major industry is leveraging COTS DQ solutions. Do you want to be the one company that falls short of industry politics?
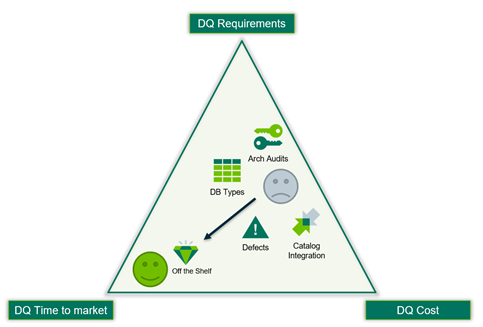
Figure 3. Accelerating time to market for your DQ solution
Given the above, one could think of many challenges to creating an asterisk in the build-vs-buy data. While these notions may hold some truth, the sum of many anecdotes and challenges does not outweigh the history of COTS prevailing – “economies of scale.” I would then leave you with one anecdote to challenge the previous.
We’re not different. Something broke – Yes something will break in Data quality. As you wait to make your decision or take the time to build your own DQ, things will keep breaking. I’ve seen DQ incidents tip into the 8 figures. So, while you weigh your decision, time is of the essence to deliver DQ. Do you really have time to wait for a team to build DQ when buying DQ is proven faster time-to-market?
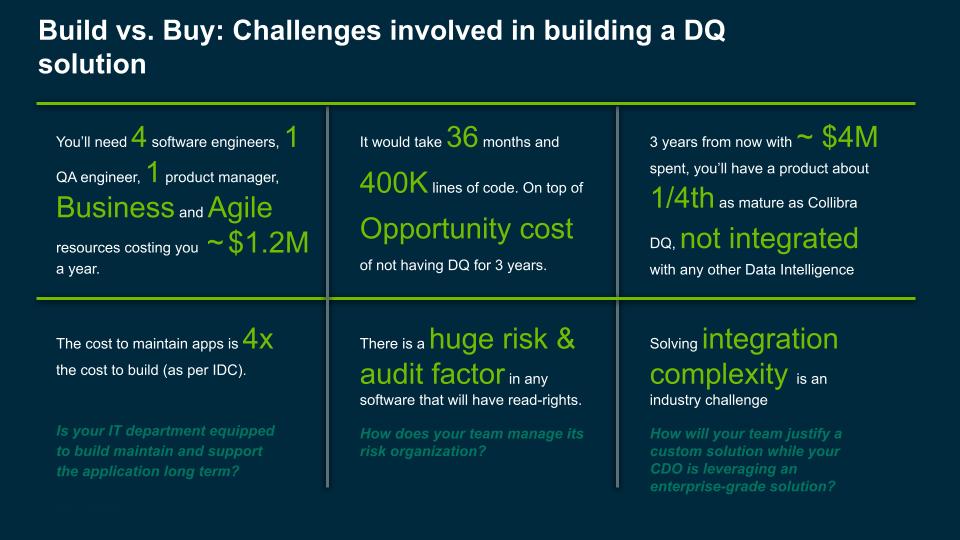
Figure 4. Challenges involved in building a DQ solution: Can you afford to wait?
The data suggests one should strongly consider buying a DQ solution built by a DQ-focused company before building their own. This should not be an existential question for development teams as there is plenty of customization data engineers can engage into to make healthy data pipelines. If you’re looking for a DQ solution that services Engineers, Operations, and Business, check out Collibra Data Quality and Observability.