Your data warehouse or data lake serves as a primary repository, fueling traditional machine learning, generative AI, AI agents, business intelligence, regulatory reporting and data products. To maximize the value of this data, it’s essential to ensure its fitness for as many use cases as possible. The reliability and trustworthiness of your AI and analytics depend heavily on high-quality data.
Without trusted and reliable data, decisions based on AI and analytics outputs will be poor, resulting in wasted resources and diminished confidence from business leaders. Implementing data quality and data observability increases trust in your data, leading to more confident and effective decisions and actions.
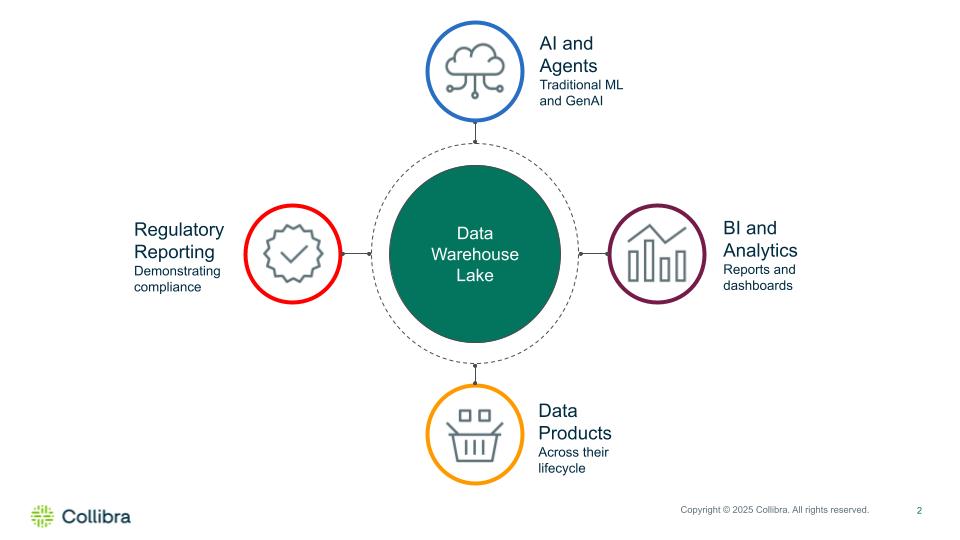
Figure 1: The data warehouse and lake are critical data repositories for AI and analytics
The quality of data in data warehouses and lakes is a top priority for AI and analytics success
While governance of data warehouses and lakes is nothing new, the increased focus on traditional machine learning and generative AI over the last few years has heightened awareness of the need to ensure high quality data.
Improving data quality and trust is the highest priority in data management for AI.
IDC, Infobrief, Closing the Gap Between Governance for Data and AI
Sponsored by Collibra and Google Cloud
April 2025 | IDC #US53252525
Whether you’re trying to better manage revenue, cost or risk with AI and analytics, poor quality data impairs your ability to make decisions and take action with confidence. Some examples of the impact of poor data quality include:
Revenue management
If the data in your data warehouse or lake is of poor quality your ability to capitalize on new revenue opportunities and address current challenges in revenue management will be compromised.
Healthcare example
The healthcare network’s data lake ingests billing and treatment data from multiple EMRs, practice management systems and external clearinghouses. However, poor data governance and quality leads to inconsistent mappings, duplicate patient records and missing procedure codes.
Common data quality challenges
- Procedure codes (CPT/HCPCS) are inconsistently formatted across facilities, leading to invalid charge submissions
- Diagnosis and procedure mismatches due to missing ICD-10 codes prevent claim processing automation
- Inconsistent patient identifiers (e.g., name variations, MRNs) prevent full visibility into treatment history and billing coverage
- Claim status data lacks standard timestamps or payer denial codes, hampering appeals analysis
Common impacts
- An excessive percentage of claims stuck in A/R > 90 days
- High manual workloads by the claims management team to reconcile claims
- The Centers for Medicare and Medicaid Services issues a notice of audit for improper payments
- Reduced cash flow creates the need for additional external financing
21% is the annual revenue growth increase realized by trailblazers as a result of invests in data and AI governance.
IDC, Infobrief, Closing the Gap Between Governance for Data and AI
Sponsored by Collibra and Google Cloud
April 2025 | IDC #US53252525
Cost management
If the data in your data warehouse or lake is of poor quality your ability to capitalize on new cost management opportunities and address current challenges with expenditures will be compromised.
Federal agency example
The agency’s procurement analytics relies on a centralized data warehouse that ingests procurement data from various departments and legacy ERP systems. However, poor data quality undermines the ability to create a reliable spend baseline.
Common data quality challenges
- The same supplier appears under multiple variations (e.g., “Acme Tech”, “ACME Technologies Inc.”, “ACME”) with no unique ID standard, masking total spend with that vendor.
- Purchase orders are missing contract identifiers making it difficult to distinguish on-contract from off-contract spend
- Items are classified inconsistently across departments (e.g., “stationery”, “admin supplies”, “general consumables”), hindering category-level aggregation
Common impacts
- Inability to capture procurement discounts from suppliers based on volume thresholds
- High manual workloads by procurement teams to reconcile and validate spend data
- Strategic sourcing decisions are delayed or misinformed
- Inability to demonstrate compliance with off-contract spend policies
- Increased oversight and scrutiny by the Office of Inspector General
20% is the annual cost reduction realized by trailblazers as a result of invests in data and AI governance.
IDC, Infobrief, Closing the Gap Between Governance for Data and A
Sponsored by Collibra and Google Cloud
April 2025 | IDC #US53252525
Risk management
If the data in your data warehouse or lake is of poor quality your ability to proactively identify and manage financial, operational and regulatory risk will be compromised.
Financial Services example
Under BCBS 239, banks are required to have strong risk data aggregation and reporting capabilities to ensure timely, accurate and complete risk reporting, especially in times of financial stress. The bank’s data warehouse pulls risk exposure data from multiple siloed systems (trading, credit, market risk). However, data quality issues arise due to inconsistent data definitions, missing metadata and lineage gaps.
Data quality challenges
- Inconsistent counterparty IDs across systems lead to duplicate or fragmented exposure records
- Data lineage is incomplete—risk reports can’t trace inputs back to source systems
- Risk metrics like VaR (Value at Risk) and exposure at default are calculated using stale or unverified data
Common impacts
- Aggregated risk exposure reports misrepresent risk due to fragmented and missing data
- After an audit the inaccuracy is discovered by regulators
- The bank faces penalties and constraints on capital distributions until compliance is remediated
- The event erodes trust and regulators increase scrutiny of the company
21% is the annual regulatory compliance improvements realized by trailblazers as a result of investments in data and AI governance.
IDC, Infobrief, Closing the Gap Between Governance for Data and AI
Sponsored by Collibra and Google Cloud
April 2025 | IDC #US53252525
Summary
Data warehouses and lakes are a primary data repository for AI and analytics. However, if the data within them is not highly trustworthy and production ready, its use can lead to flawed AI outputs, inaccurate analytics and increased business risks. Implementing data quality and observability for your data warehouse and or lake can help you ensure your AI and analytics initiatives better support management of revenue, costs and risk.
In my next blog, I will cover how to use data quality and observability to increase confidence in your data warehouse or lake. Until then, here are few resources to help you on your journey.
Want to learn more?
Watch the webinar Best practices for reliable data for AI
Read the workbook BCBS 239 workbook
Take a Product tour