Last month, I attended the AWS Capital Markets Financial Data Forum in London. With business and technology leaders from around the globe exploring how data fuels the industry, and presentations on everything related to accelerating financial data pipelines and delivering data in the cloud, the event offered executive thought leadership on emerging technologies.
Not surprisingly, everyone was talking about Artificial Intelligence (AI). With every financial services organization focused on making better and faster decisions, data professional and business leaders are eager to better understand how AI can facilitate their strategic goals. And while AI in financial services is not entirely new, the meteoric rise of generative AI in Q4 2022 dramatically increased the prevalence of AI in conversations in boardrooms across the globe.
Discussions at the event fluctuated between the recognition of the massive competitive advantage presented by AI, and the equal recognition of the risk necessitating caution and effective governance of the technology. Examples of AI missteps are being exposed daily, with companies experiencing issues with Large Language Models (LLMs) presenting invalid or fabricated results (known as hallucinations) to AI models exhibiting extreme – and entirely unwanted – bias within their results. Without effective management, AI presents a unique challenge around unintended consequences with the potential for negative business impact. Embracing an informed, measured, and governed approach to AI provides a path to maximizing ROI while helping mitigate emerging risks. The Data and AI governance experts at Collibra have been working with the AI and Cloud experts at Amazon Web Services to help customers understand the need for AI governance and implement a framework for success on AWS.
Already using AI?
Financial services orgs, especially those in capital markets, frequently has been on the forefront of generative AI investment. Customer service, risk assessment, sentiment analysis, and other use cases have all been employed to some degree within companies over the past few years. Organizations have been using Amazon SageMaker, a cloud machine-learning platform enabling developers to create, train, and deploy machine-learning models, since it was launched in 2017. A recent survey from The Economist of banking executives’ states that “85% have a “clear strategy” for adopting AI in the development of new products and services.” These models have been well studied, highly trained, and operationalized with great results. However, the rise of more broadly available generative AI capabilities has created a surge of company investment where enthusiasm and potential market advantage is minimizing the perception of risk and contributing to an increase in the potential pitfalls illustrated above. Without a mature data framework and the necessary controls the ability to understand current state, develop for the future, and properly manage a nearly continuous lifecycle of AI products or capability development is placed in jeopardy.
This recognition of the uncertainty around AI from the speakers at the conference – both from an institutional knowledge and a lack of the necessary tools and data intelligence functionality – saw frequent use of the phrase “black box.” The ability to control and evaluate company value from AI becomes increasingly difficult when data value and integrity is in question, or where a single model can proliferate into thousands overnight. As Collibra Chief Data Citizen and Co-Founder, Stijn Christiaens, recently wrote, “An AI governance framework offers a blueprint for how to create successful AI products. It is a map to a repeatable process for driving long-term, reliable AI programs.”
What does this framework look like?
With such a new and complicated subject area, Collibra has worked to develop a simple AI Governance Framework that can be used by any organization (download it here). Whether you have hundreds of models in production already or just starting to explore and implement tools like Amazon SageMaker and Amazon Bedrock, this framework can help guide you through your AI journey. Every organization anticipates to have different guidelines for the proper implementation and use of AI. With years of experience in data and AI, Collibra and AWS provides you with the flexibility to experiment with and build your AI applications, and help to develop and implement AI guidelines that are most beneficial for you.
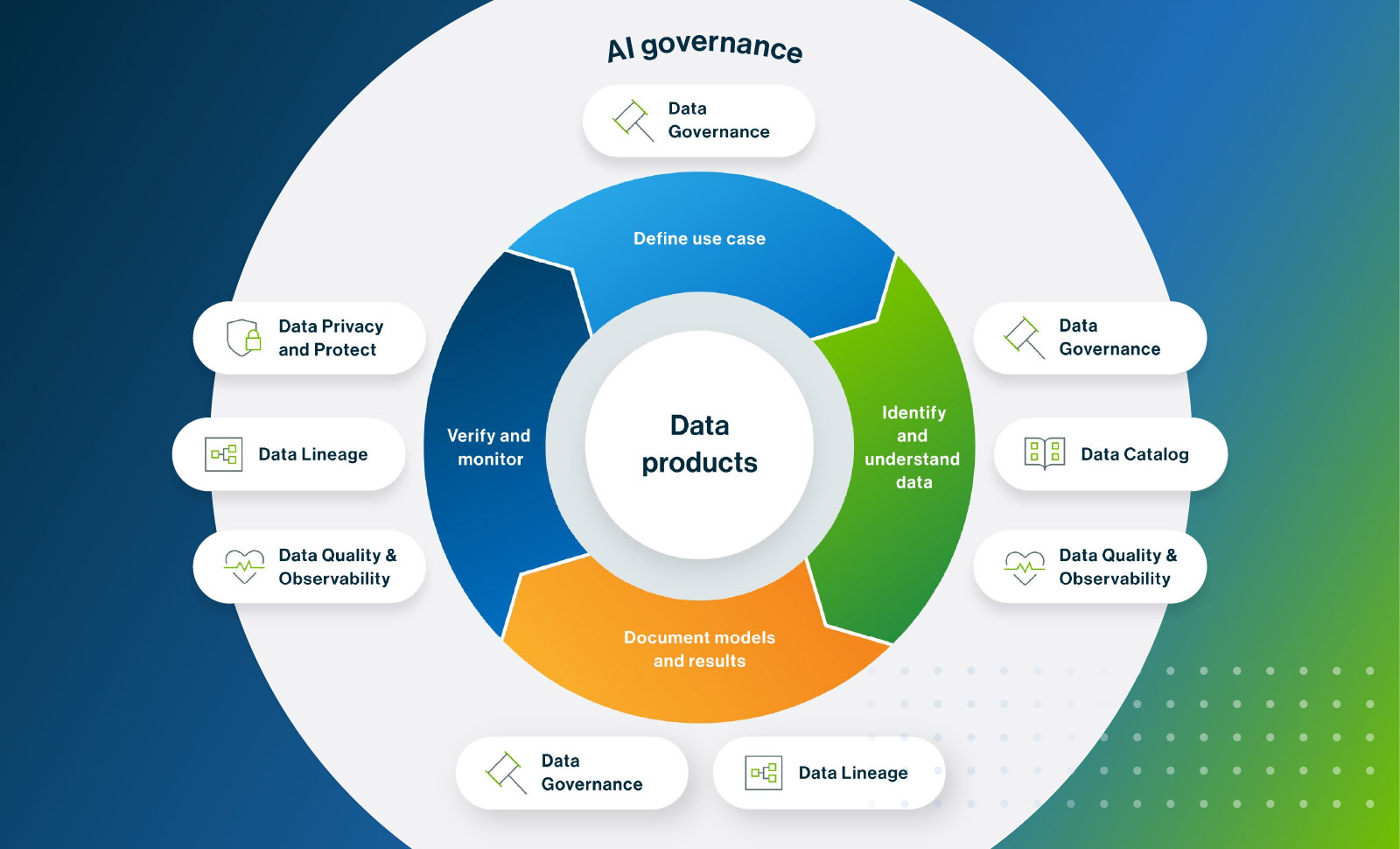
A simple but highly effective AI Governance Framework
It all starts with the use case – which AI model in Amazon Bedrock or Amazon SageMaker do you anticipate to use and how do you plan on using it? Documenting all aspects of the use case can help you explain why the model is needed and its intended purpose. This can also help decision makers, like those on your privacy and ethics teams, to weigh in to see if the model is ethical or legal to use.
Next up, you’ll want to identify and understand the data you have in order to feed the model. For example, many of the models in Amazon Bedrock and Amazon SageMaker support retrieval-augmented generation (RAG), which is a framework for improving the quality of large language model (LLM) generated responses by supplementing the LLM’s internal representation with external sources of knowledge. By using the catalog from a data intelligence platform like Collibra, you can find data, understand the data, and assess the quality of data, and know how often the data is updated.
At this point, you can then experiment with and customize the open-source models available in Amazon Bedrock, or if desired, build your own foundation model in Amazon SageMaker.
OK, your use case has been approved, you have the data you need, and you’ve customized or built your model. Now it’s time to document the model and all its results. This is where a data scientist may focus most of the project time, but the dividends are expected to be paid in the end.
Last, and certainly not least, is to continuously verify and monitor the model. Are you getting the expected results? Does the model need to be retrained? Is there a new/better model available on Amazon Bedrock or Amazon SageMaker so that the current model can be sunset? Each of your AI models has a lifecycle and when its usefulness runs out, it’s time to take it out of production. To learn more about the AI Governance framework, you can read this blog.
Mitigating Risk in AI
So, what happens when AI gets out of control and good intentions lead to bad results. To name just a few:
- Biased decision-making: If data is biased, AI can perpetuate and amplify bias, which can lead to biased (and ill-informed) decision-making.
- Inaccurate recommendations: AI models rely on patterns and correlations established by training data. If the data is flawed, inaccurate, or incomplete, then the predictive model is also unreliable.
- Outlier misinterpretation: Outliers and data anomalies can significantly impact AI models. If the AI is not trained to recognize them, then it may make erroneous, even disastrous conclusions.
- Security/Privacy risks: Poor data quality can expose sensitive information, which can inadvertently lead to security breaches and the unauthorized use of personal information.
- Legal/Ethical implications: Organizations may face legal consequences by making decisions based on inaccurate or biased AI inputs. Using AI to process personal data without adherence to privacy regulations (like GDPR or CCPA) can result in costly legal and reputational risks.
- Trust issues: Deploying AI systems that produce incorrect or biased results can erode public trust in your organization’s reputation. Investment in validation and edge case elimination are essential
Making great AI achievable
Powerful AI platforms and services like Amazon SageMaker and Amazon Bedrock provide capital markets organizations the fully managed infrastructure, tools, models, and workflows to develop AI solutions for any business use case. The Collibra Data Intelligence Cloud, with active metadata at its core, delivers trusted data for every user, every use case and across every source. Capital markets organizations harnessing the power of both AWS and Collibra have a bright future in AI ahead of them. From algorithmic trading, fraud detection, and KYC use cases, sophisticated AI models can be quickly built, verified and deployed using only the highest quality and trusted data.
Want to see it in action? Contact your local Collibra or AWS account executive today.