“With our automated world, every second thousands of decisions hinge on your data. Poor data quality doesn’t just mean mistakes—it means mistakes at lightning speed.” – Kirk Haslbeck, Founder of Collibra Data Quality, Inventor of Automated Rules
State and local governments (SLED) are leveraging AI to enhance public safety, streamline operations, and improve citizen services. As we move towards a future increasingly dominated by AI, it becomes clear that cataloging and lineage, though essential, are not sufficient on their own. The missing piece? Data Quality.
Whether you are an existing Collibra Data Intelligence Platform customer or not, adopting AI initiatives without data quality solutions in place can be disastrous. Poor data quality can lead to incorrect predictions and flawed decision-making, which can be particularly detrimental in high-stakes environments like state and local government (SLED).
For example, consider a SLED use-case where AI models are employed to predict and manage traffic flow in smart cities. Accurate data is crucial for these models to function effectively. Poor data quality can lead to incorrect predictions, causing traffic jams or even accidents, undermining public trust in government technology initiatives. Thus, ensuring high data quality is not just about maintaining data integrity—it’s about safeguarding public safety and trust.
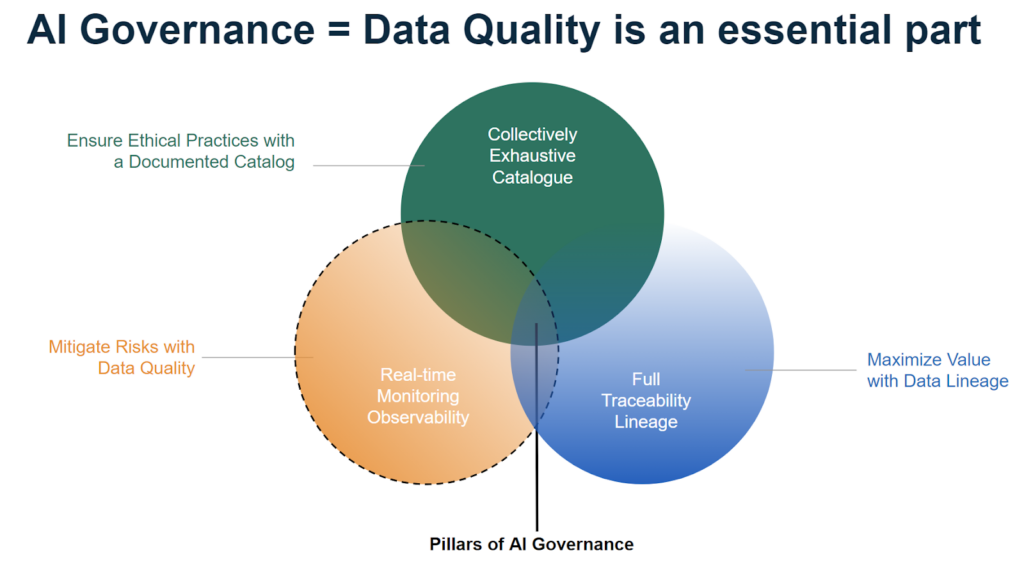
Integrating Data Lineage, Catalog, and Quality – The Pillars of AI Governance
Collibra Puts ML/AI to Work to Make Your AI Work
Collibra leverages machine learning (ML) and artificial intelligence (AI) within its Data Quality & Observability (DQ&O) solutions to improve the reliability and accuracy of your AI models. This means that Collibra can manage and monitor your data pipelines, identify and support rectifying anomalies, and ensure that the data feeding into your AI systems is of the highest quality. By automating these processes, Collibra allows you to focus on the more custom and strategic aspects of AI relevant to your specific industry or business use case.
Here’s How Collibra Data Quality & Observability is Indispensable for AI:
- Seamless Data Connectivity and Efficient Job Execution: In the realm of AI, particularly with large language models (LLMs) and complex AI systems, the ability to seamlessly connect to diverse data sources is crucial. Collibra Data Quality supports over 40 databases and file systems, ensuring that data can be efficiently scanned and validated where it resides. This capability is especially beneficial for platforms like Databricks, Snowflake, and Google Big Query, where pushdown processing optimizes performance and scalability, ensuring that data is ready for AI model training and inference without bottlenecks.
- Adaptive Rules with AI-generated Insights: AI systems must adapt to dynamic data environments where trends and data patterns shift rapidly. Collibra’s AI-generated Adaptive Rules create thousands of monitoring controls in minutes, automatically adjusting to new data trends. This adaptability ensures that data anomalies are detected and corrected in real-time, preventing the propagation of bad data into AI models, which is critical for maintaining model accuracy and reliability.
- Automated Data Classifications: For AI governance, particularly in sensitive applications like healthcare and finance, automated data classification is vital. Collibra leverages industry-specific rules to classify and enforce data quality automatically. This ensures that AI models are trained on clean, compliant data, reducing the risk of bias and enhancing the model’s decision-making capabilities. Automated enforcement of data quality rules ensures consistency and reliability across the organization.
- Generative AI for Data Quality Rules: Crafting complex data quality rules can be a time-consuming task, but Collibra’s generative AI simplifies this process. By using natural language prompts, users can quickly generate and customize data quality rules. This acceleration in rule creation allows for rapid deployment of data quality measures, ensuring that AI models are always working with the most accurate and relevant data, enhancing their predictive power and robustness.
- Monitoring for Schema Changes: AI models are highly sensitive to changes in data structure. Collibra continuously monitors schema evolution and detects unexpected schema drifts. This proactive monitoring prevents potential issues that could affect AI model performance, such as data misalignment or incorrect feature extraction. By ensuring that data schemas remain consistent, Collibra helps maintain the validity of AI outputs, providing a stable foundation for AI-driven insights and decisions.
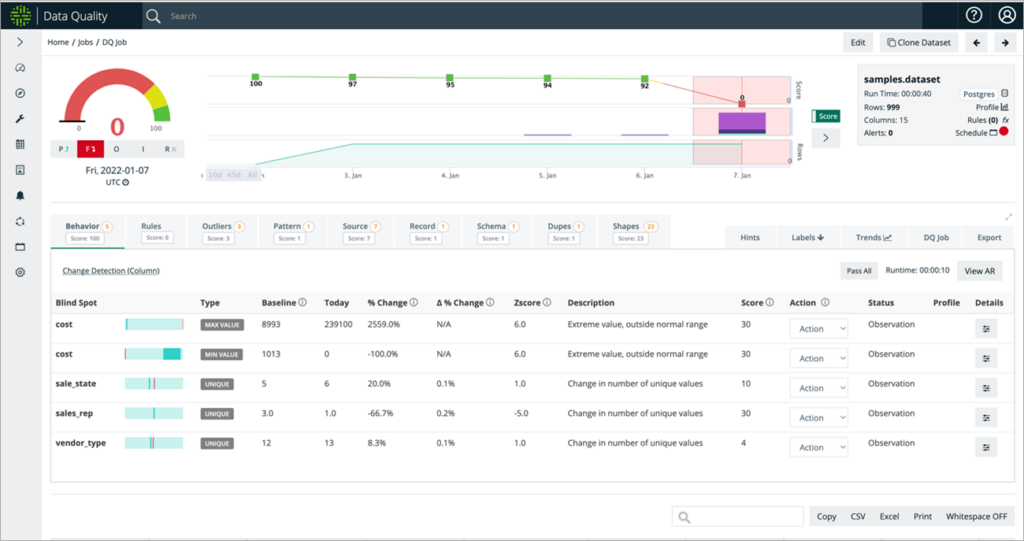
Features for Robust AI Governance – Maximize Data Integrity, Ensure Ethical Practices, and Mitigate Risks.
Your Comprehensive System for Data Engagement
In conclusion, while cataloging and lineage are crucial, adding data quality into the mix ensures that the data is not just well-documented but also reliable and trustworthy. This integration is vital for robust AI governance, helping organizations maximize the value of their AI initiatives while maintaining ethical standards and compliance.
To learn more, check out the Collibra Data Quality & Observability page for more information, free trials, and quick product overviews to see the product in action.