Before a chess match begins, there is order; the board is symmetrical, with all the pieces lined up in their rightful spots. The queen and king stand proudly in the center of the board, guarded on either side by the knights and bishops. The front line of pawns provide the initial shield to the queen and king. While this initial layout is orderly and methodical, as soon as the first player makes their move, chaos ensues. After the first move, there is no more order. Rather, after the first move, every other move has a direct impact on the outcome of the next move. In fact, after both players take their first move, 400 games exist. After the players make their second move, 197,742 games exist. And after the players take their third move, 121 million games exist. This pattern continues until the game is finished, with an estimated 10^120 possible games according to mathematician Claude Shannon. This impressive number of games is greater than the number of observable atoms in the universe and grains of sand on Earth!
Similar to chess where every move impacts the next, a small physical change to data storage or movement can impact the flow of data upstream and downstream. As a business user, it is important that you are aware of these changes since they could dramatically impact reports you use to make business decisions. It is also important for IT to perform impact analysis before they make any changes to guarantee the changes do not negatively affect business. With Collibra Lineage, you can do this automatically. Our automated data lineage capability enables the enterprise to conduct impact analysis at a granular level (columnar level or a table or business report). This automated process saves data engineers valuable time over manual analyses.
Conducting impact analysis with a data lineage solution
Without Collibra Lineage, organizations must spend countless hours manually conducting impact analyses. In fact, to create one impact analysis, data engineers must go through five key steps that take multiple hours, or even days, at a time:
- Data engineers must go into their company server and dig up spreadsheets with manually mapped data lineage.
- Since finding the right spreadsheet is often difficult, data engineers typically need to go around to their colleagues and different departments within the organization and ask for help.
- Once they find the spreadsheet they are looking for, they must check back in with the owner of the spreadsheet to determine if it is up to date. Since it is created manually, most likely the spreadsheet is outdated and incorrect.
- As a result, data engineers must go into the spreadsheet and start from scratch; they must check over every relationship and remap the data.
- Finally, data engineers must manually go through every line of code in the spreadsheet (sometimes thousands of lines) to see how changing one line of code impacts the rest of the data flow.
These five steps all occur before data engineers can even begin analyzing the impact that a change to a data source or system can have. Not to mention, manually conducting impact analyses can negatively affect a business. If data stakeholders are unaware of changes to their data, they could use inaccurate reports to make important business decisions. Thus, it is crucial that the organization does impact analyses in a timely manner to avoid detrimental effects of critical business decisions being based on bad data.
Why Collibra Lineage works for impact analysis
When IT makes a change to data and data pipelines, it is imperative that data engineers alert stakeholders of the change immediately so that they can prepare appropriately. It is also important for data engineers to understand and recognize the impact of the change on every data set and endpoint in the system; one little change can affect more than just that one particular data point. Because of this, it is crucial that companies invest in an automated data lineage solution to effectively and efficiently conduct impact analyses.
Unlike creating a manual impact analysis, which takes at least five steps and numerous hours, with Collibra Lineage, data engineers can see the impact that any change will have within seconds. You simply click into your technical lineage view to visually see the relationship between your data.
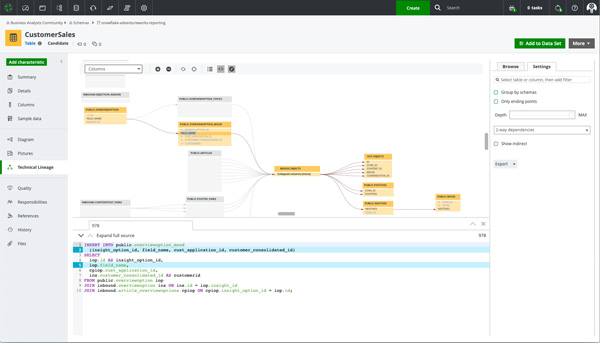
Collibra Lineage illustrates these relationships through arrows that connect the tables and columns. This visualization enables you to see the impact your change has on every other data point within the company’s system. If you want to learn more, you can drill down into relevant table and column-level code that provides more context. With the code you can see all the details about how data flows in and out of the columns. With all this information, IT can be proactive and alert stakeholders of any changes ahead of time.
Winning the game
Every move in chess impacts the next. Humans spend their whole life trying to predict their opponents’ next move in a game. This is truly an impossible task. In fact, in 1996 the computer, Deep Blue, defeated Gary Kasparov, the world champion of chess, by predicting Kasparov’s every move. This revolutionary match up illustrates the value of impact analysis. In this game, Deep Blue automatically conducted an impact analysis after every one of Kasparov’s turns. This enabled Deep Blue to stay ahead of Kasparov in the game.
This match between Deep Blue and Kasparov shows the importance of automated impact analysis. The manual moves of Kasparov were no match for the automated intelligence of Deep Blue. Collibra Lineage enables you to win the match. It gives you the tools and knowledge to create impact analyses effectively and efficiently. While manual impact analysis takes at least five steps, countless hours, and numerous people, with Collibra Lineage you can gain the information needed for an impact analysis with a few clicks. The automation allows IT to focus on strategic initiatives instead of manually mapping data and ensures the business user is using the right data to drive business decisions. This enables IT to stay ahead of confusion and alert data stakeholders before they use their data incorrectly.